Hindering the Expansion of Problematic AI
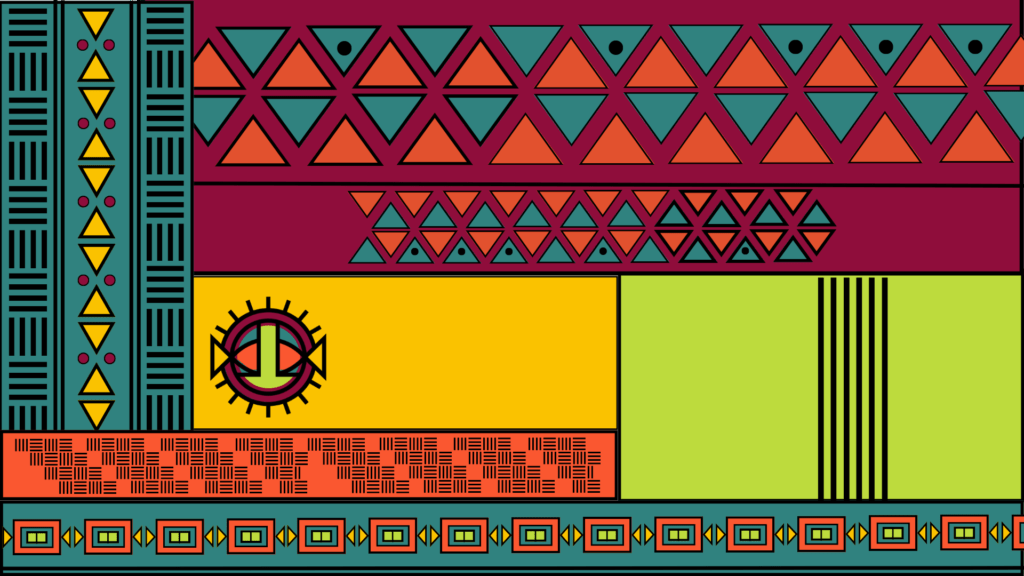
I am an engineer by training three times. The third time was in Japan. On one September my father came to visit me and we had many fascinating conversations. By far, one of my favourite subjects was his theory of expansion. He doesn’t call it that. He may not even recall the conversation we had but the theory of expansion states that:
“Like the Universe’s overwhelming tendency to expand, so too does everything living in it seek to mimic that expansion”
Goloatshoene Moiloa.
He went on further to say that humans were special because they do not merely just expand in their physical form but in their mental capacity as well. It is out of this desire to expand that AI continues to evolve to meet the limits of the human imagination. It also, inadvertently ventures outside of it.
The Problematics
We have many examples of this inadvertence. In 2020 alone advanced technologies were behind: britain delaying effective Covid19 spread prevention methods, a Telegram bot app that removed the clothing off pictures of women, “universal” technical tools like Twitter and Zoom perpetuating racial discrimination and language models being praised for their ability to smash certain academic benchmarks when their real life applications continuously prove to be a threat to already discriminated, marginalised, oppressed and vulnerable communities (read: bot suggests suicide to imitation mentally ill patient).
Solutions under development
These and many other stories have brought the dangers of AI into the consciousness of users and creators of AI. Experts concerned with the field have formalised academic pursuits to assist in better understanding the issues at play. Three points of intervention commonly identified (at the FACT conference) are the:
- Machine Conceptualisation (MC) phase which deals with problem definitions of things we try to solve
- Machine development (MD) phase which deals with technicality around conducting analysis
- Machine release (MR) phase which deals with best practises of developmental release as well as policy development
For the MC stage primary concerns are around the ethics of the problems we are trying to solve and the metrics we deem fit to represent them. Also under scrutiny at this stage are the resources that motivate the pursuance of a particular agenda. This includes sourcing the right data and understanding under what circumstances the data is true and relevant.
Concerns within the MD phase include the secretive nature of most of the algorithms we utilise to make decisions. It includes how certain assumptions and biases regarding particular problems are hidden within these secrets. These are addressed with tools that aim to demystify the black box. (see this Github Repo)
In the MR phase, concerns are twofold. Both in the release of datasets and models into the open source world to be used and re-used in different contexts. But also in the development of best practise, policy and other regulatory strategies to better manage AI’s unintended outcomes. (see the EU Commission Proposal for the Regulation of AI)
Is it enough?
It is assumed that we would have dodged any major threats of pending doom by AI if we cover our bases in these three areas. Mitigation techniques are important to incorporate but they fail to address the fact that machines function within the hegemony. Any machine developed within the realm of the hegemony lies subject to its rules and practices. Machines that follow these practices serve the status quo. And most likely at the expense of someone or something else.
Mind you, this hegemony is established in an AI born of; a history of war, modern statistics originating from eugenics, commodifying people and their information and scientific principles founded upon rationality and functionalism which both seek to identify observed phenomena and mechanisms of the world as independent and modular, logical and reasonable. Which means referencing individualistic western ideologies as the base of the humanhood we wish to mimic in our machines. This singular view violates generally speaking, indigenous ways of knowing but more importantly indigenous ways of being — Ways of knowing and being founded upon the eternal pursuance of unification.
The problem is us
The unintended impact of our machines comes as no surprise when we understand the underlying origins they embody. A technology is incapable of tending to the diversity of paradigms that the technology is meant to serve when it has embedded in it harmful points of view. We also realise that if we want AI to be good, then the people developing it need to be. And at the very least, developers need to be capable of understanding the limitations of their perspective.
A large part of being “good”, is being held accountable for the points of view we hold and pass onto our machines. This is done in part by expanding our understanding of the reality of different narratives. But Building “good” AI also means expanding its founding principles to involve advanced practices. It involves developing practices that do not center AI development around people who already benefit from the status quo but instead centers those who stand to be harmed by it. It means expanding the function of AI beyond individual profit and capital gain. And allowing it the space to function in a world that is not logical and within reason.
We need a shift
The push for Machine driven decision making is motivated by a desire for insight into the world. We want to know how best to navigate it. And to better understand opportunities for getting out of it what we want. Through AI our imagination for wanting has been expanded beyond anything we ever thought we might’ve guessed. But with increased opportunities for procuring newly imagined wants and the excitement surrounding the possibility of their materialisation as quickly as possible means that understanding whether these wants and their methods of realisation are good or bad is a complicated process given different contexts.
Shifting the direction of this expansion is a process that requires us to consider the careful steps to take with these technologies on our journey into executing a successful human-machine hybrid future. It also requires considering how the history of the technology itself has shaped the way in which we think about what it is that we want and what we are willing to lose to get it. The universe expands in a manner that is chaotic, let us not be tempted to replicate it’s chaos…